Breakthrough in earthquake prediction Using Machine Learning
In a significant advancement for earthquake prediction, a team of researchers from the Department of Information Technology at Georgia Southern University has unveiled a new machine learning and neural network model that has achieved an impressive accuracy rate of 97.97% in forecasting seismic activities in Los Angeles, California. This pioneering study builds upon prior research, establishing a strong foundation for utilizing advanced machine learning techniques to enhance earthquake prediction accuracy.
The researchers focused on optimizing predictive capabilities by employing a meticulously crafted feature matrix integrated with the Random Forest algorithm, which proved to be the most effective among 16 different machine learning algorithms tested. According to Professor Lei Chen, a co-author of the research, this innovative approach offers invaluable predictive tools that could assist authorities in better preparing for seismic risks and hazards.“The application of sophisticated machine learning algorithms such as Random Forest, combined with neural networks, has enabled us to achieve groundbreaking results in seismic forecasting,” remarked Professor Yiming Ji, another co-author of the study. The team's findings not only expand the horizons of earthquake prediction but also establish a framework for future advancements in predicting other natural disasters.Previously, the researchers had achieved a 69.14% accuracy rate in predicting the maximum earthquake magnitude within six categories. They later expanded their research efforts to Istanbul, Turkey—an area known for its seismic activity—achieving a commendable 91.65% accuracy rate, and further improved this to 98.53% in San Diego. Building on these successes, the team returned to Los Angeles to refine their model, culminating in a remarkable 97.97% accuracy rate.Cemil Emre Yavas, another co-author of the study, highlighted the significance of these results: “Our model’s 97.97% accuracy represents a substantial leap forward from traditional methods, offering crucial insights that could save lives and mitigate property damage in high-risk zones.”To develop their predictive model, the researchers leveraged earthquake data from the Southern California Earthquake Data Center (SCEDC), managed by the California Institute of Technology, analyzing reports spanning the past 12 years. They focused on identifying deep seismic patterns and testing a range of tools, aiming to enhance earthquake prediction accuracy significantly.The study utilized a radius of 100 km (62 miles) around Los Angeles to encompass a broad area relevant to earthquake forecasting. This careful selection helps ensure that critical seismic events are included in the dataset while minimizing noise from regions with differing seismic characteristics.Through rigorous fine-tuning of hyperparameters, the Random Forest model was able to deliver robust and precise predictions. This finely tuned model not only facilitates deeper analysis but also serves as a vital tool for earthquake forecasting.The research builds on a comprehensive array of earthquake prediction studies conducted between 1990 and 2024. The advancement of neural network models, such as the graph convolutional neural networks explored by Bilal et al., showcases the potential of sophisticated technologies to improve predictive accuracy.Furthermore, initiatives like the Collaboratory for the Study of Earthquake Predictability (CSEP) and the Regional Earthquake Likelihood Models Experiment (RELM) have laid the groundwork for breakthroughs in earthquake prediction. Diverse data sources, including GPS and ionospheric data, have contributed to the evolution of forecasting models, exemplified by Gitis et al.'s work on seismological data and Zhai et al.'s exploration of thermal anomalies.This research represents a significant step forward in earthquake prediction, offering promising avenues for enhancing public safety and emergency response strategies in the face of seismic threats.
In a significant advancement for earthquake prediction, a team of researchers from the Department of Information Technology at Georgia Southern University has unveiled a new machine learning and neural network model that has achieved an impressive accuracy rate of 97.97% in forecasting seismic activities in Los Angeles, California. This pioneering study builds upon prior research, establishing a strong foundation for utilizing advanced machine learning techniques to enhance earthquake prediction accuracy.
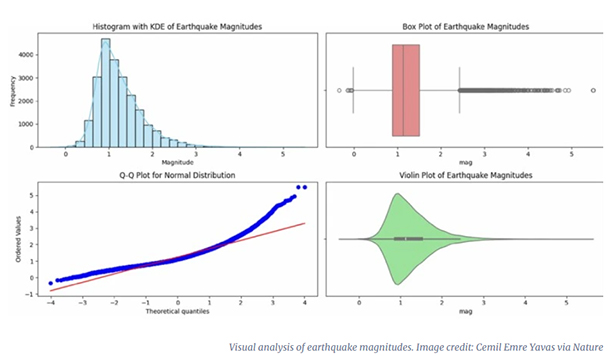